Retrieval-Augmented Generation - The Future of AI is a Hybrid Approach
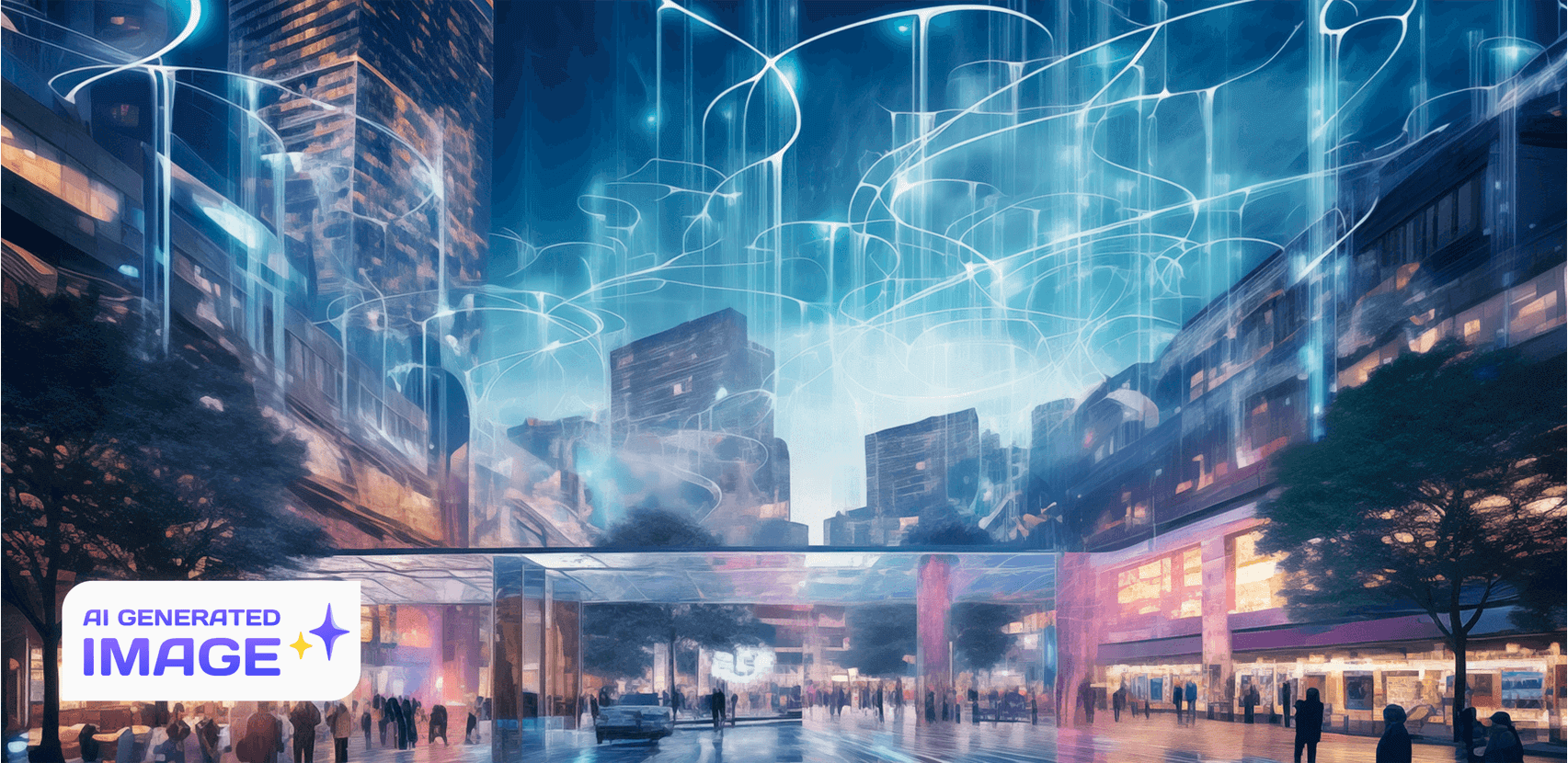
Artificial intelligence has advanced rapidly within just a few years, but systems like OpenAI still face limitations in accuracy and relevance in delivering responses. What if AI could leverage both its existing knowledge and access the most up-to-date information, may it be the internet or any data-bearing systems? This is where Retrieval-Augmented Generation or RAG comes to life!
In this article, we’ll learn together what RAG really is, how it works, its advantages, and what are its current use-cases in the expanding AI landscape.
Retrieval-Augmented Generation Explained
In 2020, a paper published by Patrick Lewis together with a team of Facebook AI researchers suggested a concept that would drastically improve the responses of generative AI systems — Retrieval-Augmented Generation or RAG.
RAG is an approach to enhance generative AI models’ accuracy and reliability in query responses by providing supplementary knowledge through context and data that may or may not exist on its current knowledge base. It’s basically adding data/knowledge to a prompt to get a more concise and factual response.
The principle of RAG is to optimize a large language model’s (LLM) outputs using specified data without having to alter the existing LLM itself. The specified data mentioned can be the most updated information of what the LLM (ChatGPT, Claude, Bard, etc.) is already trained on, adding emphasis to certain points, and can refer to literally anything. This approach allows the generative AI system to provide more definite and appropriate answers to questions.
The Process of How Retrieval-Augmented Generation (RAG) Works
RAG is currently the best-known tool for grounding LLMs on the latest, verifiable information. To grasp its essence, let’s take a closer look at the step-by-step process of how RAG really works:
- User enters prompt — It begins when a user provides the specific query.
- Augmentation — The AI system now starts augmenting prompts with data that aligns with the objectives and intent of the user. Though this varies significantly between systems as it could include internet search, SQL queries, user data supplementation, etc.
- Generation — After augmentation, the LLM returns a generated response to the user based on the enhanced prompt.
Advantages of the RAG Approach
Implementing RAG in LLMs has an evident benefit: It ensures that the model has access to the most current, reliable facts, and that its claims can be checked for accuracy and ultimately trusted.
RAG techniques can be used to improve the quality of a generative AI system’s response to prompts, beyond what an LLM alone can deliver. Benefits include:
- Enhance accuracy — Responses to user queries will be more relevant and up-to-date than just relying on generative AI’s existing knowledge.
- Better at aggregating information — RAG can aggregate data from numerous sources and generate fresh human-like responses. This is essential for complex queries requiring data from multiple sources.
Current Use-Cases of the RAG Approach
AI technology continues to flourish, and it’s not showing any signs of slowing down. Like any other technology, the RAG approach is yet to be perfected, which means improvements will be evident with endless possibilities. At the moment, businesses of all sizes are maximizing the use of AI in their workflows. Let’s dive into some real-life examples of how the RAG approach is utilized:
- Providing comprehensive data to user queries to get more targeted responses.
- Including domain specific proprietary knowledge to improve the relevance of a response.
Takeaways
Early RAG approaches have already demonstrated impressive results, but this is just the beginning. As techniques advance, we can envision RAG powering truly assistive AI. Immense future possibilities await!
However, there are still challenges to overcome. Knowledge aggregation, reasoning integration, multi-question answering, and controllable generation remain areas of research. As researchers and developers improve RAG techniques, we inch closer to AI that realizes its full potential.
What is certain is that RAG has opened up an exciting new paradigm in AI system design. By combining extensive data retrieval and extensive context generation, we can create solutions greater than we can ever imagine!
Sources
- https://blogs.nvidia.com/blog/what-is-retrieval-augmented-generation
- https://research.ibm.com/blog/retrieval-augmented-generation-RAG
- https://www.promptingguide.ai/techniques/rag
- https://www.oracle.com/artificial-intelligence/generative-ai/retrieval-augmented-generation-rag/
- https://www.cohesity.com/glossary/retrieval-augmented-generation-rag/
- https://searchengineland.com/how-search-generative-experience-works-and-why-retrieval-augmented-generation-is-our-future-433393
More of Our Starship Stories
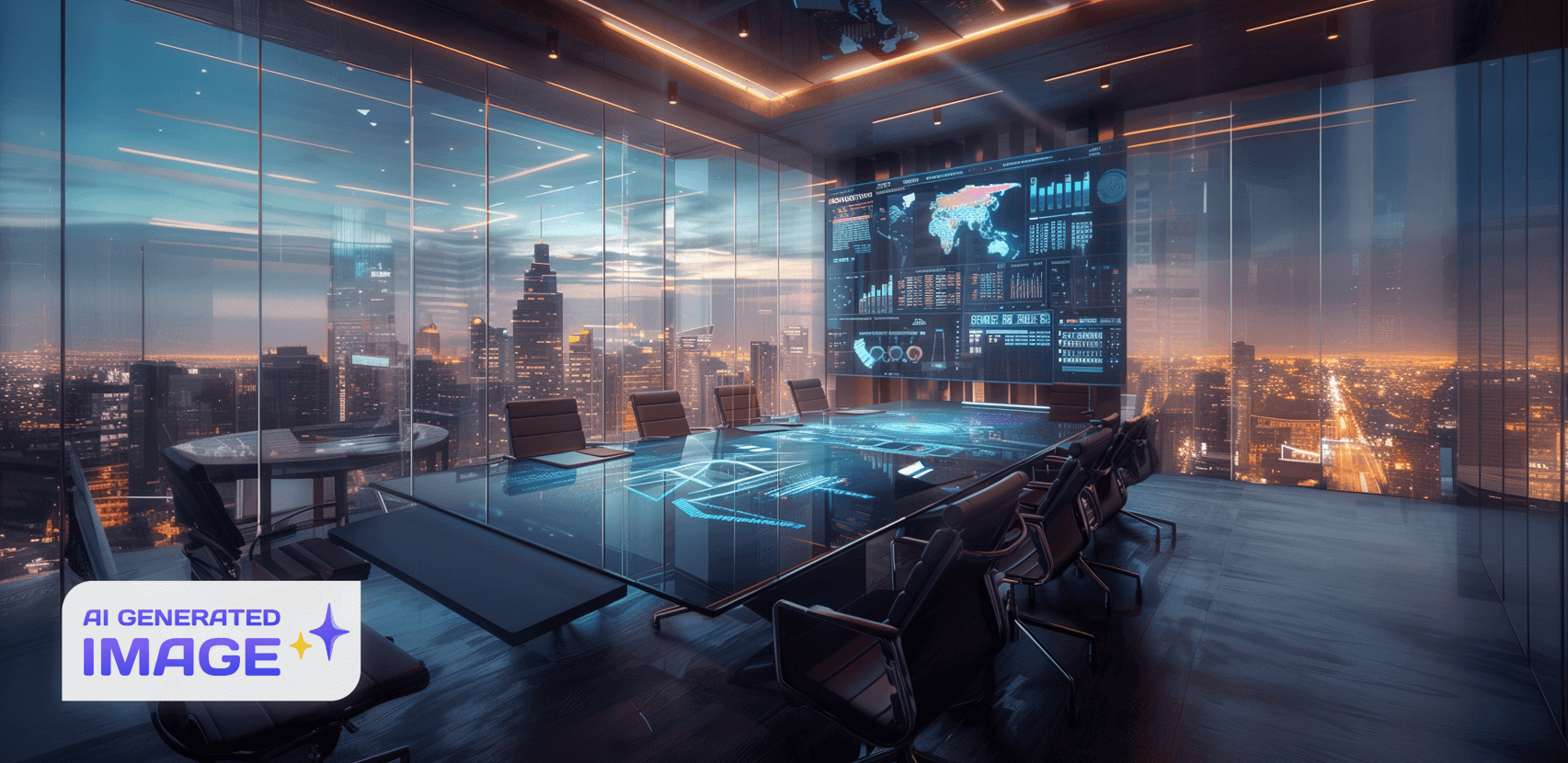
How to Build a Financial Model For a Startup
October 10, 2024
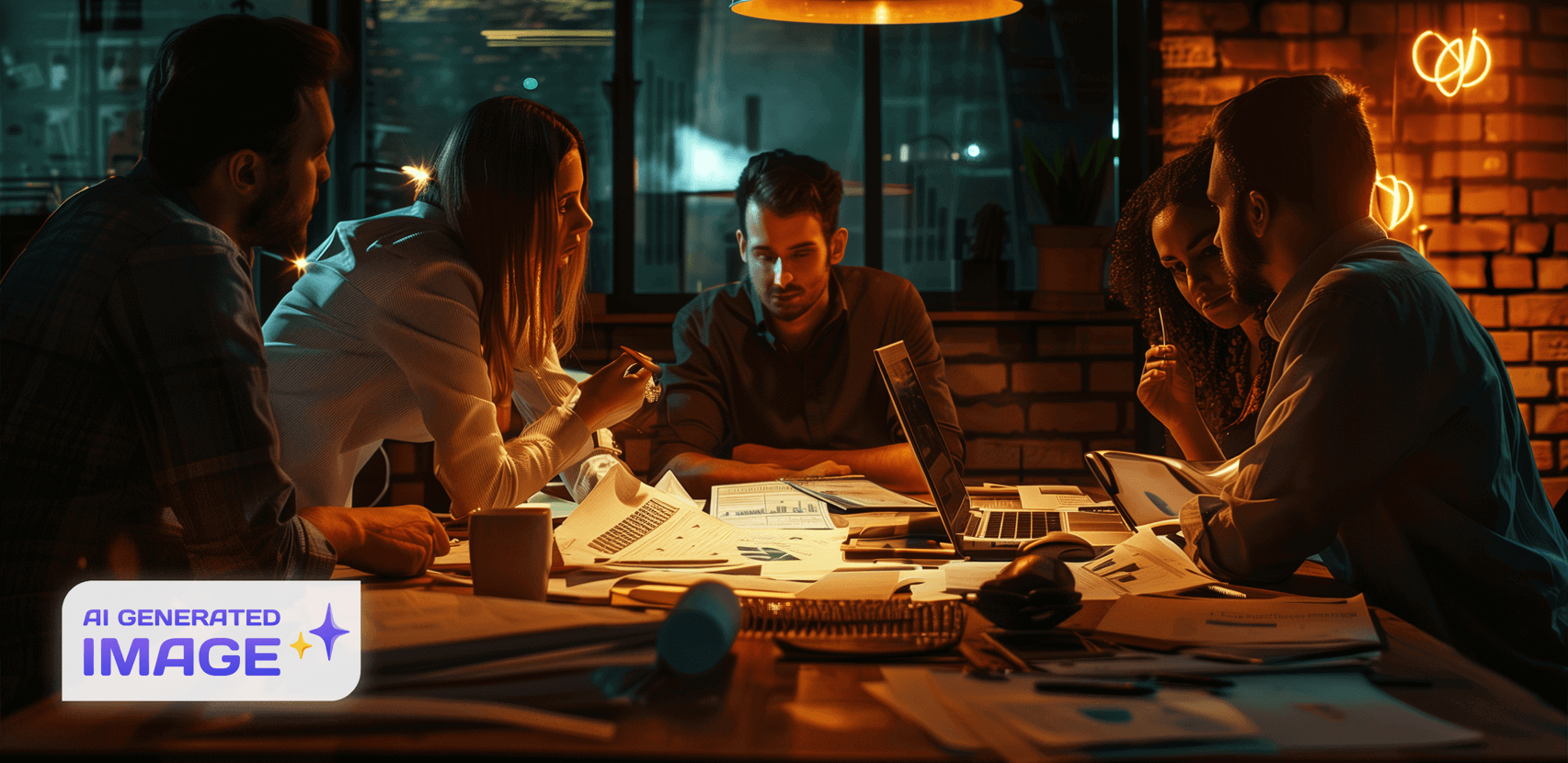
Why Your Early Stage Startup is Failing
September 23, 2024
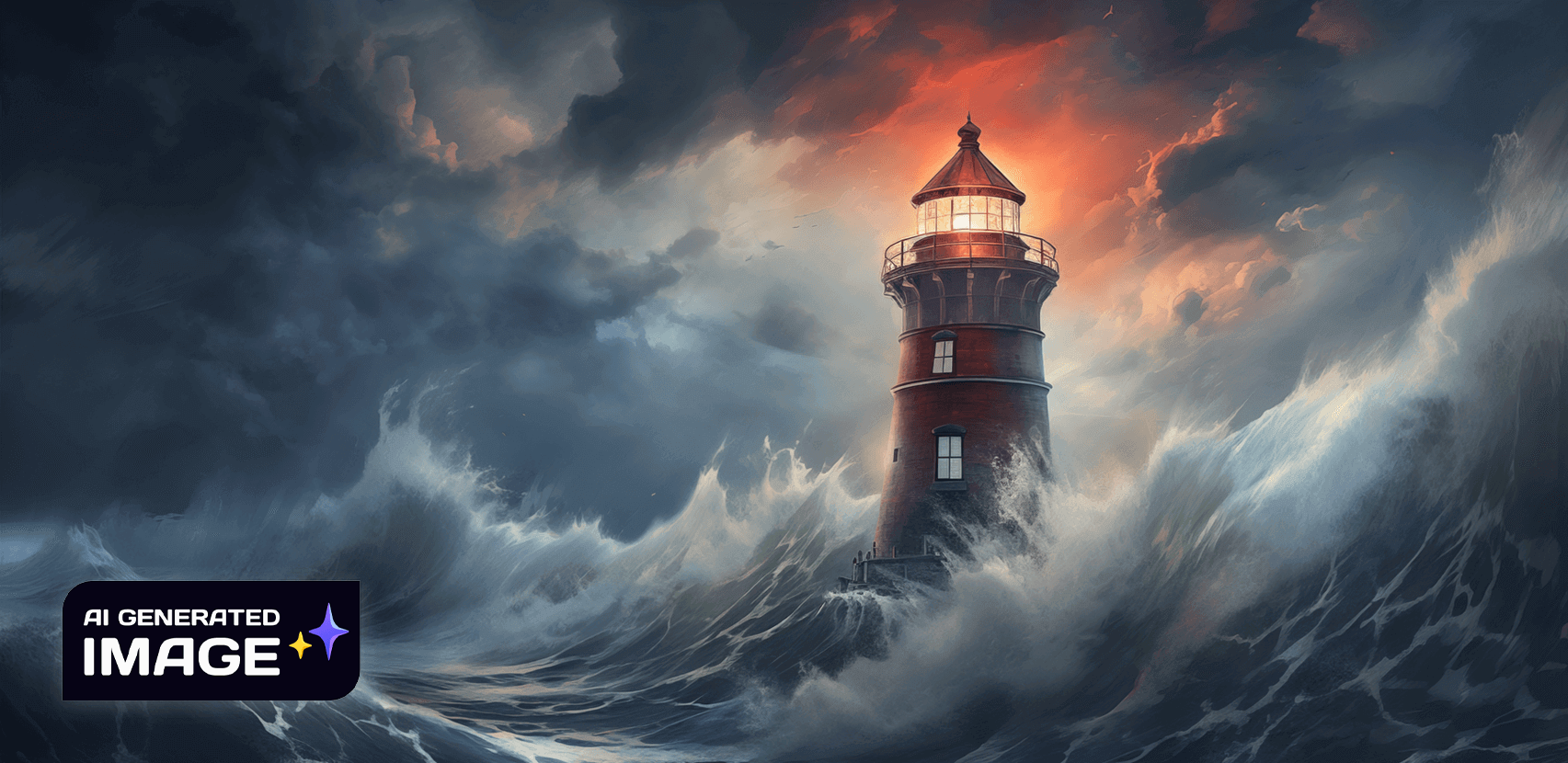
Should a Startup Hire a CTO?
January 3, 2024